The International Medical Device Regulators Forum (IMDRF) has released its final guidance document (IMDRF/AIML WG/N88:2025) outlining Good Machine Learning Practice (GMLP) for medical device development. This document provides 10 key guiding principles to ensure the safe, effective, and high-quality development of artificial intelligence (AI)-)-enabled medical devices, particularly those using machine learning (ML) algorithms.
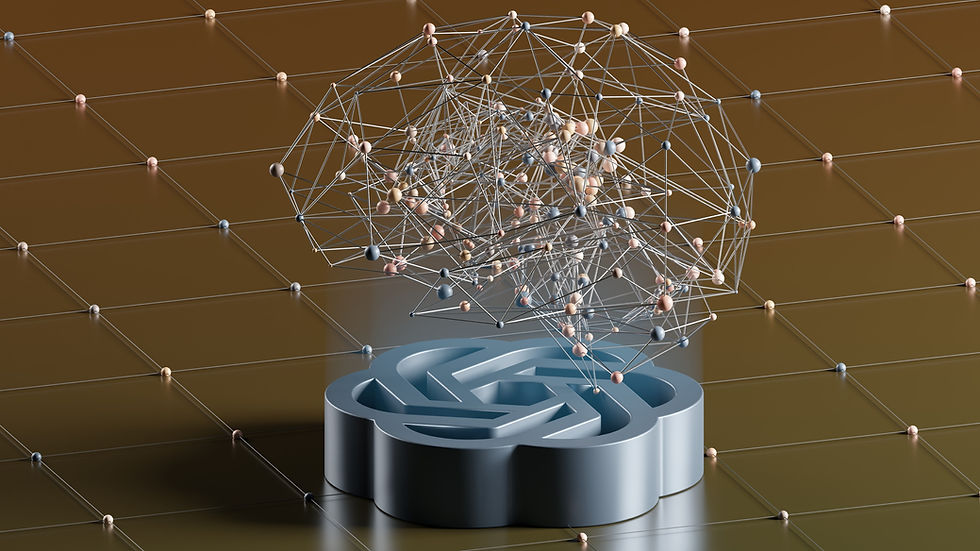
With AI and machine learning playing a growing role in healthcare, this guidance aims to establish a common global standard, ensuring that these technologies are safe, reliable, and ethically designed.
AI, particularly machine learning (ML), has transformed healthcare by continuously allowing medical devices to learn from vast amounts of data. Unlike traditional static algorithms, ML models can adapt and improve over time, offering enhanced accuracy and predictive capabilities. However, this dynamic nature also introduces regulatory challenges related to safety, performance validation, and clinical effectiveness.
The IMDRF’s GMLP principles serve as a global framework to support the development and regulating of AI/ML-enabled medical devices. These principles aim to ensure that AI-based medical technologies are:
Safe and effective for their intended use.
Developed using best practices in software engineering and clinical evaluation.
Continuously monitored to prevent unintended bias, performance drift, or cybersecurity risks.
The 10 Guiding Principles of GMLP
1. Well-Defined Intended Use Throughout the Product Life Cycle
Understanding the intended use of the device is critical. Developers must define:
The clinical workflow in which the AI-enabled device will be used.
The patient population the device is intended for.
The expected benefits and risks associated with its use.
By integrating multi-disciplinary expertise (AI engineers, clinicians, regulators), developers can ensure that AI-enabled medical devices meet clinical needs and maintain patient safety.
2. Strong Software Engineering, Medical Device Design, and Cybersecurity Practices
AI-driven medical devices must be designed, tested, and maintained with rigorous software engineering principles, including:
Robust risk management frameworks.
Secure data handling and privacy protections.
Cybersecurity protocols to prevent unauthorized access and data breaches.
3. Representative Data for Clinical Evaluation
Clinical evaluation should use diverse datasets that accurately represent the intended patient population. This includes considering:
Age, gender, ethnicity, geographic location, and medical conditions.
Dataset size and quality to ensure generalizability and prevent biases.
Addressing dataset drift to maintain model accuracy over time.
4. Separation of Training and Test Datasets
To ensure accurate validation, training datasets should be completely independent from test datasets. This prevents overfitting and ensures the AI model can generalize well in real-world scenarios.
5. Fit-for-Purpose Reference Standards
Developers must use clinically relevant reference standards for model validation. These should be based on:
Established medical benchmarks and regulatory consensus.
Documented rationale for their selection.
Continuous assessment to determine whether they remain valid over time.
6. AI Model Choice and Design Based on Intended Use
Machine learning models must be carefully designed and tested to mitigate risks such as:
Performance degradation over time.
Security vulnerabilities.
Incorrect predictions leading to adverse clinical outcomes.
Additionally, the model’s explainability should be considered—transparent AI improves trust among healthcare providers and patients.
7. Focus on Human-AI Interaction in Clinical Environments
AI-enabled devices should be assessed not just in isolation, but in real-world clinical workflows, where healthcare professionals and patients interact with them. Key aspects include:
Human factors and usability testing.
Training for healthcare providers on AI model interpretation.
Avoiding over-reliance on AI predictions by clinicians.
8. Performance Testing in Real-World Conditions
AI medical devices must be tested in clinically relevant conditions, with:
Controlled and uncontrolled variables.
Subgroup analyses to identify populations where AI performance may vary.
Independent validation datasets to simulate real-world performance.
9. Clear and Transparent Information for Users
Manufacturers must provide clear documentation to users (healthcare professionals or patients) regarding:
Intended use and limitations of the AI model.
Performance metrics across different patient populations.
How the AI model integrates with clinical workflows.
Known risks and potential errors.
10. Continuous Monitoring and Risk Management
AI medical devices must have post-market surveillance mechanisms in place to detect:
Performance drift over time.
Bias in model predictions.
Software updates that could alter clinical performance.
If the model undergoes retraining or updates, manufacturers should ensure:
Robust version control.
Risk assessment for every update.
Regulatory approvals where necessary.
Comentarios